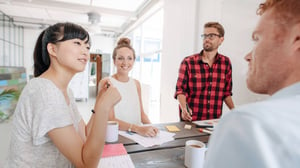
Synthetic Data Improves Microsoft’s Next-Gen Conversational AI Engine
At a glance
Spur Reply helped Microsoft create better modeling within structured, synthetic data production that resulted in an evolving, scalable conversational artificial intelligence (AI) engine. Our collaboration improved functionality performance, increased data collection relevancy, reduced annotation overhead, and expanded production capacity.
Business results
Services provided
Data strategy, engineering, and analysis
AI/machine learning
Content strategy and development
Project, process, and program management
SCHEDULE A MEETING WITH AN EXPERT
Microsoft’s next generational and conversational AI engine
The quantity of usable data and the quality of deep learning algorithms determine how “smart” an AI engine can become. However, most ventures reach the end of their data supply long before their algorithms begin to falter.
Microsoft, a leader in technology advancements, acquired Sematic Machines to serve as its next-generation AI engine. The AI needed to be trained on practical technologies for accurate responses with the end-users. To drive this result, Microsoft decided to study the AI’s ability to calendar by scheduling relevant meetings, reading daily agendas, and responding to similar tasks, allowing the tech company to focus on four components:
- Improving data quality and product performance
- Building a tailored data set focused on mitigating identified gaps and training their AI
- Streamlining data production processes and defining best practices
- Developing strategies to focus data production on accelerating product development
Barriers to AI engine development
Microsoft sought Spur Reply’s expertise to innovate their data production processes and systems. The technology leader asked us to help improve the data quality and acceptance of its highly sophisticated conversational AI engine.
Furthermore, limited data production scale failed to accelerate product and feature development, and the lack of available data hindered the AI engine’s deep modeling and machine learning components.
The outsourced data production needed higher quality and relevancy standards. Minimal training in data across key scenarios additionally created gaps in the AI knowledge base.
Refining the synthetic data production process
Our team developed a strategy for tackling large-scale data production with Microsoft. We aided the client in recruiting the right talent, building the culture, and developing the execution strategy.
“Our partnership significantly accelerated the evolution of our AI engine and our progress toward our product vision,” said Mikko Ollila, Microsoft Principal Program Manager.
Within two weeks of strategy development, our team established a team of data scientists to audit Microsoft’s data production, workflow, processes, systems, and algorithms. Taking a hands-on approach, we spent the initial phase working side-by-side with Microsoft’s team to fully understand the end-to-end workflow. We considered existing processes and uncovered gaps in the data.
Once completed, we pivoted our focus to working in lockstep with AI researchers to define gaps in the knowledge base and response generation. The targeted data enabled our team to create 56 classifications and predictions to increase the conversational AI responses. Subsequently, we developed a Markov chain algorithm to provide automatic language categorization.
Improved data management and process optimization
Quantitative results show we successfully improved Microsoft’s data management. Our teams raised average functionality performance by 14%. While managing AI performance across more than 100 product features, we additionally performed 36 data migrations to ensure data usability and model acceptance.
In terms of process optimization, our team saved thousands of hours in data production through machine teaching and automation strategies. We built automated solutions to reduce manual annotation requirements by up to 96%. Our teams also helped increase data collection relevancy and reduced annotation overhead by 33%. Finally, we expanded production capacity by training an additional 18 specialists and 12 annotators in conversational AI best practices and processes.
We would not be in the position we are today without Spur Reply. They brought a level of expertise and professionalism that was above and beyond.
Related Content
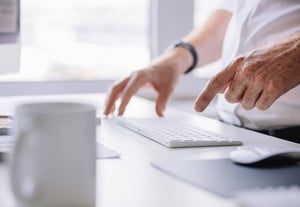